Behavioral Economics and Data Science: Turning Big Data Into Human Insights
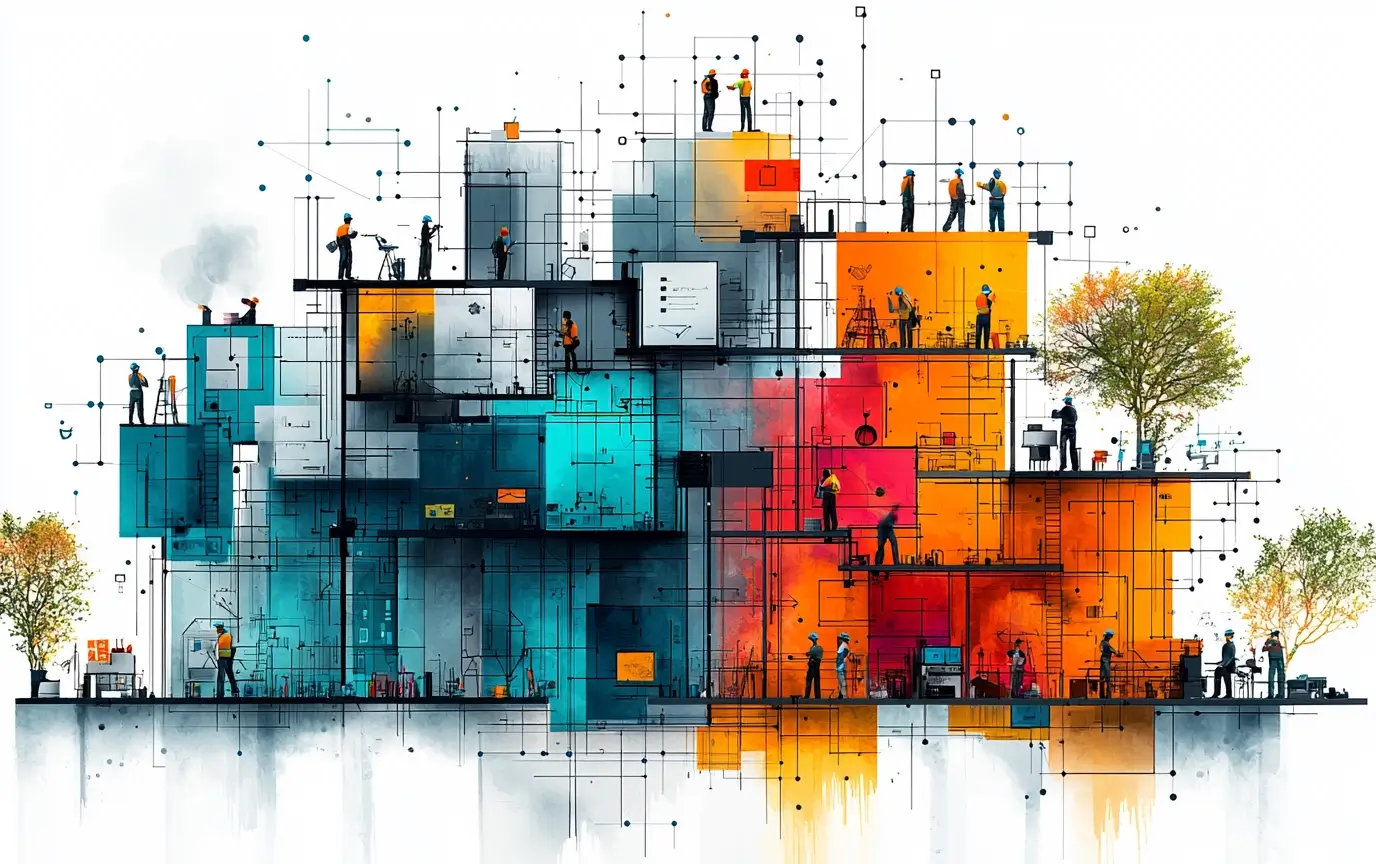
In the rush to collect data, many companies forget one thing: people aren't spreadsheets. Behind every data point lies a decision—why someone clicked, hesitated, complained, stayed, or churned. And while data science can show us patterns, it rarely explains why those patterns exist. That’s where Behavioral Economics steps in.
When combined, Behavioral Economics and Data Science become a powerhouse. One shows us what people are doing; the other explains the irrational, emotional, cognitive reasons behind it. This fusion transforms analytics from observation into understanding—from dashboards to decisions that work with human nature, not against it.
In this article, we’ll explore how organizations can use Behavioral Economics to decode their big data, apply insights to customer and employee experiences, and create human-centric systems at scale. Let’s dive in.
1. Why Data Alone Isn’t Enough
Big data tells you what’s happening. But it often misses why. You might know:
- Where customers are dropping off
- Which channels underperform
- What employees click or ignore
But without Behavioral Economics, you’re only seeing outcomes, not motives. You won’t know if the drop-off happened because of friction, ambiguity, social proof failure, cognitive overload, or default bias.
For example:
- A customer abandoning checkout might be experiencing choice overload
- A survey with low response rates could be triggering reactance bias
- An employee ignoring training reminders might be overloaded by present bias
Data alone won’t tell you this. But Behavioral Economics will.
You can’t optimize human behavior until you understand human behavior.
2. Behavioral Economics: Making Data Human
Behavioral Economics adds depth to data science by embedding psychological context into datasets. It transforms metrics into meaningful actions.
Here’s how it works:
- You observe a behavior via data (e.g., 40% drop-off at step 3)
- You hypothesize a behavioral mechanism (e.g., decision fatigue)
- You design an intervention (e.g., simplify choices, nudge copy)
- You test and validate the change using data again
This is how platforms like Amazon or Netflix personalize—not by numbers alone, but by understanding what behavior the data reflects: sunk cost fallacy, familiarity bias, social proof.
The most advanced organizations now combine:
- Quantitative analytics (clicks, churn, time on page)
- Behavioral mapping (decision trees, emotional friction points)
- Experimental design (A/B testing based on biases, not random UX guesses)
The result? Predictive empathy—you not only predict what users will do but understand why they do it.
3. The Data Science Stack Needs a Human Layer
Most data science stacks include:
- Data collection
- Data warehousing
- ETL (Extract, Transform, Load)
- Analytics engines
- Machine learning
But what’s missing is a human cognition layer—a model that accounts for attention, memory, emotion, and decision shortcuts. Behavioral Economics brings that layer.
Let’s say your churn model is 86% accurate. Great. But does it help you reduce churn? Not unless you know:
- What decisions are driving that churn
- How friction, uncertainty, or trust are influencing that behavior
- What cognitive nudges could prevent it
That’s the gap Behavioral Economics fills.
For example:
- In telecom, churn prevention improved by 31% when behavioral cues were applied to retention offers (e.g., loss aversion framing: “Don’t lose your accumulated loyalty benefits”).
- In government services, Renascence helped a client reduce drop-offs in application forms by simplifying language and applying goal gradient theory, resulting in a +40% completion rate.
These aren’t tricks—they’re data-informed, human-centric designs.
4. Behavioral Segmentation: Beyond Demographics
Traditional segmentation sorts users by:
- Age
- Gender
- Income
- Region
But Behavioral Economics pushes us to segment by decision patterns, such as:
- Cognitive load tolerance: Do users abandon when too many choices are present?
- Trust sensitivity: Do they hesitate on unclear terms or legalese?
- Action-orientation: Do they respond better to urgency or social validation?
Renascence uses Behavioral Archetypes, where users are clustered based on biases, habits, and motivation styles. This is especially powerful in industries like education, health, or banking—where users share the same demographics but behave wildly differently.
Imagine knowing not just who your customer is—but how they think when making decisions.
That’s the value of Behavioral Segmentation.
5. The Role of Cognitive Biases in Data Patterns
One of the most underutilized tools in modern data science is the bias lens—the ability to look at a data pattern and ask: Which cognitive bias is shaping this behavior?
Let’s look at a few examples where bias creates clear, trackable effects:
- Default Bias: When users stick with pre-selected options (insurance opt-ins, donation settings, etc.). Data might show a 90% uptake, but the reason is inertia, not persuasion.
- Loss Aversion: Users are 2–3 times more likely to act to avoid losing something than to gain something. Framing data patterns through this lens explains why certain offers succeed even if they aren’t the “best deal.”
- Social Proof: High conversion on product pages with reviews isn't just about product quality—it's the presence of social cues. Without recognizing this, a data team might misattribute success to price.
Understanding these biases doesn’t just explain what happened—it helps predict how people will respond to changes in wording, layout, timing, and context.
At Renascence, we've applied this principle in redesigning feedback systems. For example, when an education client wanted to increase parent response rates to satisfaction surveys, we reframed requests to emphasize what others had already done (“8 out of 10 parents in your group have completed this—your voice matters too”). Response rates increased by over 30%, purely through behavioral reframing.
The insight? Data is rarely neutral—it reflects deeply human cognitive tendencies.
6. Predictive Modeling with Behavioral Variables
Traditional predictive models use structured variables: age, tenure, income, purchase history. But by adding behavioral variables, companies can drastically improve accuracy and interpretability.
Let’s compare:
Without Behavioral Input
- Prediction: This user may churn
- Reason: They haven’t logged in for 7 days
With Behavioral Input
- Prediction: This user may churn
- Reason: Their last experience triggered ambiguity bias, and they didn’t complete a progress milestone
By layering models with:
- Nudge responsiveness
- Response to scarcity
- Delay aversion
- Trust signals clicked
…organizations create emotionally intelligent models, which not only predict behavior but suggest how to intervene.
In the Middle East, Renascence supported a government platform by enriching churn models with behavioral flags. Instead of simply analyzing click-path drop-offs, the team assessed cognitive friction at each step and deployed behavior-specific interventions (e.g., visual progress bars, pre-filled defaults, social norm statements). Completion rates jumped by over 25% without needing to add new incentives or policies.
The takeaway? Behavioral signals are predictive accelerators.
7. Turning Insights into Nudges
Once behavioral patterns are revealed through data, the next step is designing nudges—small environmental or informational shifts that guide better decision-making without removing freedom.
Types of nudges include:
- Visual hierarchy nudges: Making the most beneficial option larger or more central
- Timing nudges: Sending reminders right when motivation peaks
- Framing nudges: Using loss vs. gain language strategically
- Commitment nudges: Asking users to pre-commit to an action
Nudges are most effective when they are:
- Subtle but intentional
- Aligned with user goals
- Embedded in the environment—not forced
Data science helps us identify where nudges are needed, while Behavioral Economics helps us understand how to design them.
Example: In one CX project, Renascence used nudge design to increase feedback participation in retail settings. By presenting a short form that opened with “Help others like you have better visits,” and showing a completion bar at the top, submission rates improved by 44%—without offering any financial incentive.
Data guides placement. Behavioral science shapes influence.
8. Challenges in Merging Behavioral Economics with Data Science
Despite its value, the fusion of behavioral economics and data science isn’t without hurdles:
- Lack of Behavioral Expertise: Many data teams are fluent in statistics, not psychology. Without behavioral context, correlations are often misinterpreted.
- Overfitting Without Meaning: Models may find strong patterns (e.g., people click more on Fridays), but fail to understand why, limiting usefulness in strategy.
- Ethical Design Risks: Behavioral nudges can be manipulative if not transparently and ethically applied.
- Siloed Teams: In most organizations, behavioral teams and data science units are disconnected, preventing integration of methods and insights.
Overcoming these challenges requires:
- Cross-disciplinary teams
- Behaviorally trained analysts
- Transparent experimentation
- Ongoing ethical reviews
At Renascence, our behavioral technologists often act as the translation layer—helping data scientists understand how cognitive mechanisms shape behavior, and how to model those nuances into prediction and design.
Merging disciplines isn’t optional anymore—it’s the path to meaningful, ethical insight.
9. Behavioral Dashboards: Visualizing More Than Metrics
A powerful way to merge data science and behavioral economics is through behavioral dashboards—analytics interfaces that don’t just show counts, but show cognitive context.
These dashboards might include:
- Bias hotspots (where drop-offs suggest overload or mistrust)
- Nudge performance (which messages moved behavior)
- Emotional triggers (based on survey and interaction data)
- Decision friction scores (based on task completion, search, and abandonment rates)
This transforms insights from “25% of users dropped off at Step 4” to “Step 4 triggers ambiguity bias due to legal jargon—recommend rewriting and pre-approval nudge.”
Such dashboards are emerging in large service platforms, healthcare portals, and HR systems—turning cold data into empathetic diagnostics.
Behavioral dashboards don’t replace traditional analytics—they elevate it.
10. Use Cases: Where the Fusion Creates Real Value
Let’s ground this in reality. Here are five verified use cases where the fusion of behavioral economics and data science produces meaningful outcomes:
- CX Personalization in Loyalty Apps
- Behaviorally tagged segments (impulse buyers vs. planners) guide personalized nudges.
- Data + behavior = increased repeat visits and reward redemptions.
- Employee Learning Platforms
- Present bias and default aversion are embedded into content release pacing.
- Nudges based on behavioral cues increase course completion rates.
- Digital Government Services
- Friction mapping in forms guides redesigns that reduce abandonment.
- Behavioral cues (e.g., progress bars, social norms) improve compliance.
- Healthcare Appointment Systems
- Scarcity and effort bias are used to drive timely booking.
- Behavioral reminders reduce no-shows by up to 20%.
- Banking Onboarding Journeys
- Loss aversion and goal gradients improve conversion and reduce form drop-off.
- Trust design (e.g., secure framing, clarity) impacts sign-up behavior.
In each of these, the power came not from data or behavior alone—but the fusion of both.
11. Tools and Teams Needed to Make It Work
To fully integrate behavioral economics into data science, organizations must build:
- Cross-functional squads: Pair behavioral designers, analysts, and product owners.
- Behavioral data models: Tag data with cognitive flags (e.g., choice density, timing, abandonment)
- Toolkits: Use platforms like Renascence’s Rebel Reveal to diagnose bias and design interventions
- Ethical review systems: Ensure nudges are empowering, not manipulative
This is where Behavioral CX and EX design systems—like those Renascence has deployed in government, education, and retail sectors—become more than journeys. They become dynamic, data-fed behavioral ecosystems.
The future of data is not just automated—it’s aware.
12. Final Thought: Making Data Feel
Data without context is noise. Behavior without measurement is guesswork. But when you combine the precision of data science with the empathy of behavioral economics, you get something powerful: insight that feels human.
We no longer need to choose between being smart and being emotionally intelligent. We can build systems that understand people not just as users or employees, but as flawed, brilliant, irrational, emotional humans—and design for them accordingly.
That’s how you turn Big Data into Better Decisions. And Better Decisions into Better Experiences.
Check Renascence's Signature Services
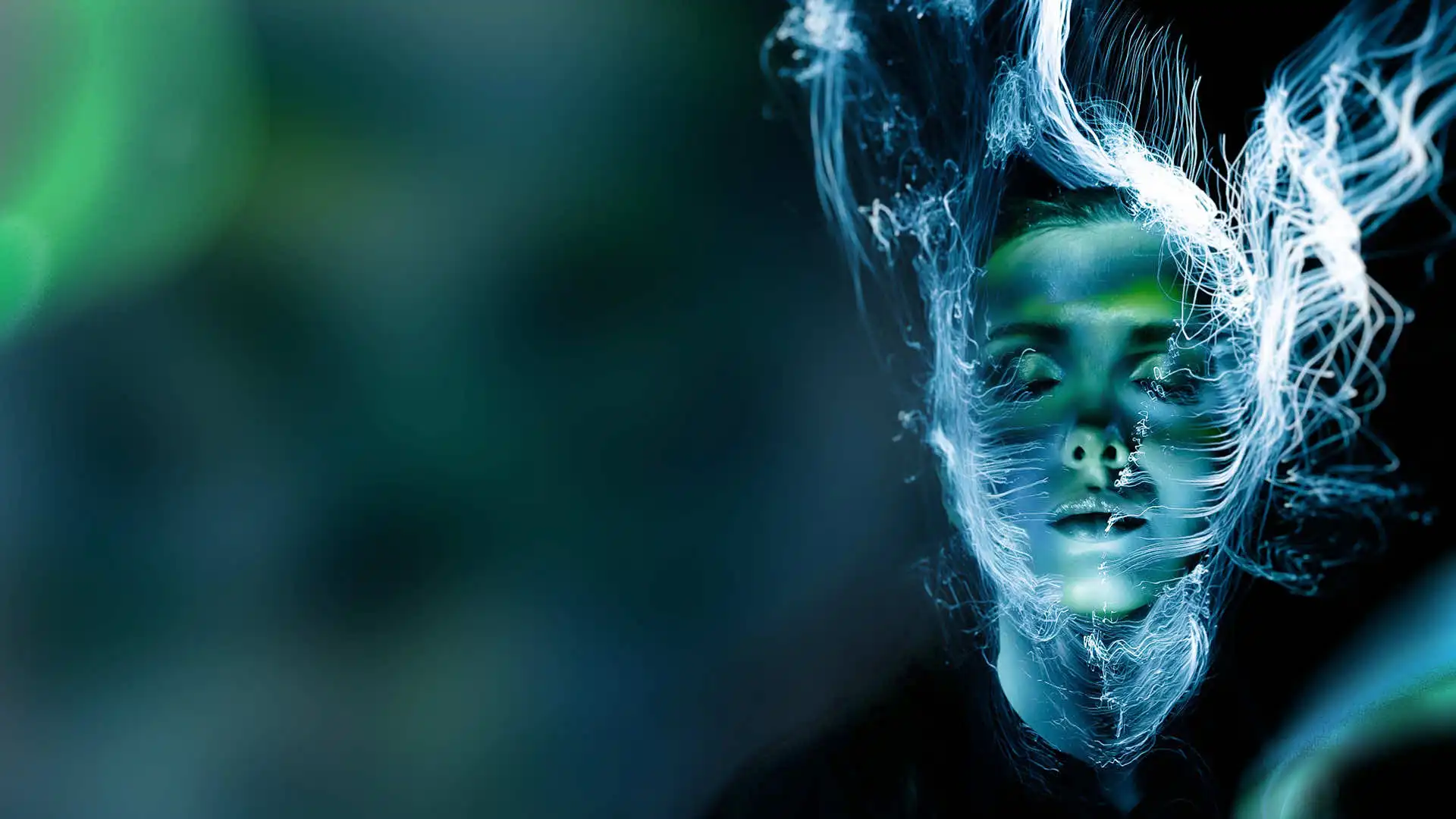
Behavioral Economics
Discover the power of Behavioral Economics in driving customer behavior.

Mystery Shopping
Uncover hidden insights with our mystery shopping & touchpoint audit services.
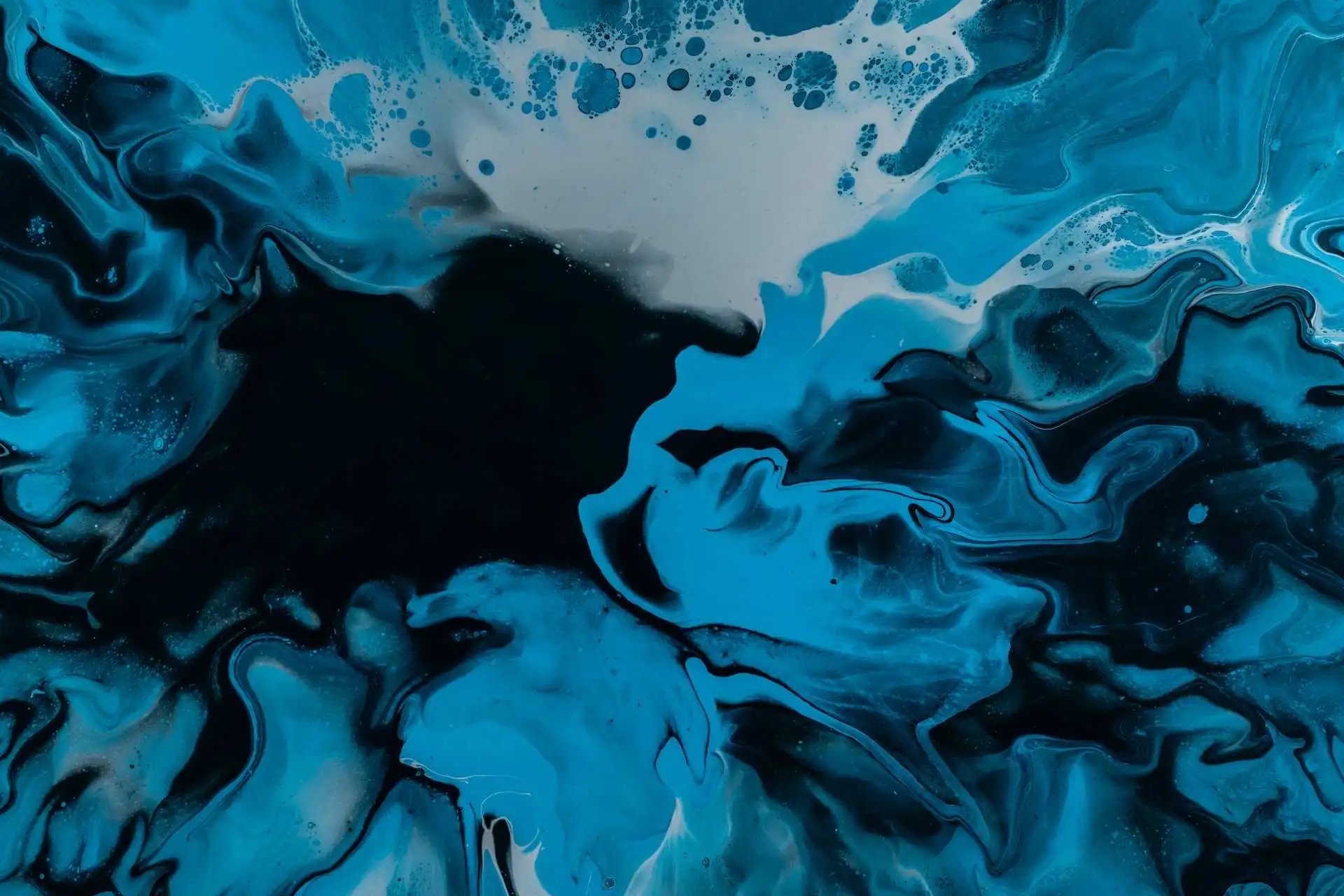
Experience Design
Crafting seamless journeys, blending creativity & practicality for exceptional experiences.
Experience Loom
Discover the latest insights from industry leaders in our management consulting and customer experience podcasts.
The Naked Customer. Episode 3. Customer Loyalty, Brand Loyalty & CX
In this episode of The Naked Customer Podcast, host Aslan Patov is joined by JD Ackley, CEO of RAIZOR AI, to explore the evolving role of AI in customer experience (CX) and its impact on automation, job security, and creativity.
In this episode of The Naked Customer Podcast, host Aslan Patov is joined by Mark Hamill, CEO & Co-founder of ARCET Global, to explore the evolution of Customer Experience (CX) and its growing role in business transformation.
In this episode of The Naked Customer Podcast, host Aslan Patov is joined by Mark Hamill, CEO & Co-founder of ARCET Global, to explore the evolution of Customer Experience (CX) and its growing role in business transformation.
In this episode of The Naked Customer Podcast, host Aslan Patov is joined by JD Ackley, CEO of RAIZOR AI, to explore the evolving role of AI in customer experience (CX) and its impact on automation, job security, and creativity.
Experience Journal's Latest
Stay up to date with our informative blog posts.
%20in%20Healthcare.%20A%20Cure%20for%20Patient%20Pain%20Points.webp)
Customer Experience (CX) in Healthcare: A Cure for Patient Pain Points
%20Trends%20in%202026.%20What%20to%20Expect.webp)
Digital Transformation (DT) Trends in 2026: What to Expect
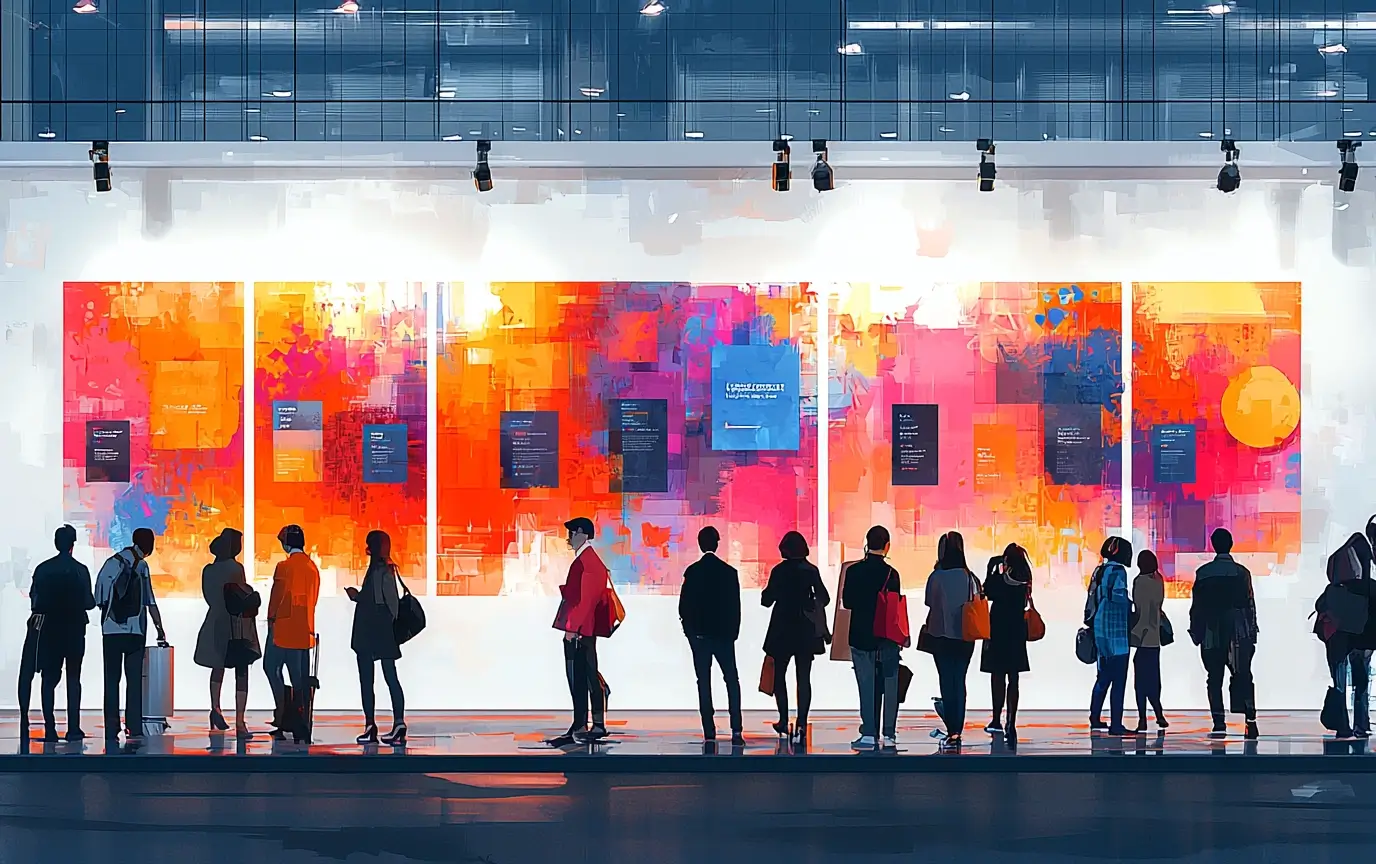
Behavioral Economics for Business: How Companies Use It Every Day
%20How-To.%20Practical%20Tips%20That%20Work.webp)
Employee Experience (EX) How-To: Practical Tips That Work
.webp)
The Critical Factors Influencing Employee Experience (EX)
%20Jobs.%20How%20To%20Succeed%20in%202025.webp)
Remote Employee Experience (EX) Jobs: How To Succeed in 2025
%20for%20SMEs%20in%20the%20Middle%20East.%20What%20Works%20and%20What%20Fails.webp)
Customer Experience (CX) for SMEs in the Middle East: What Works and What Fails
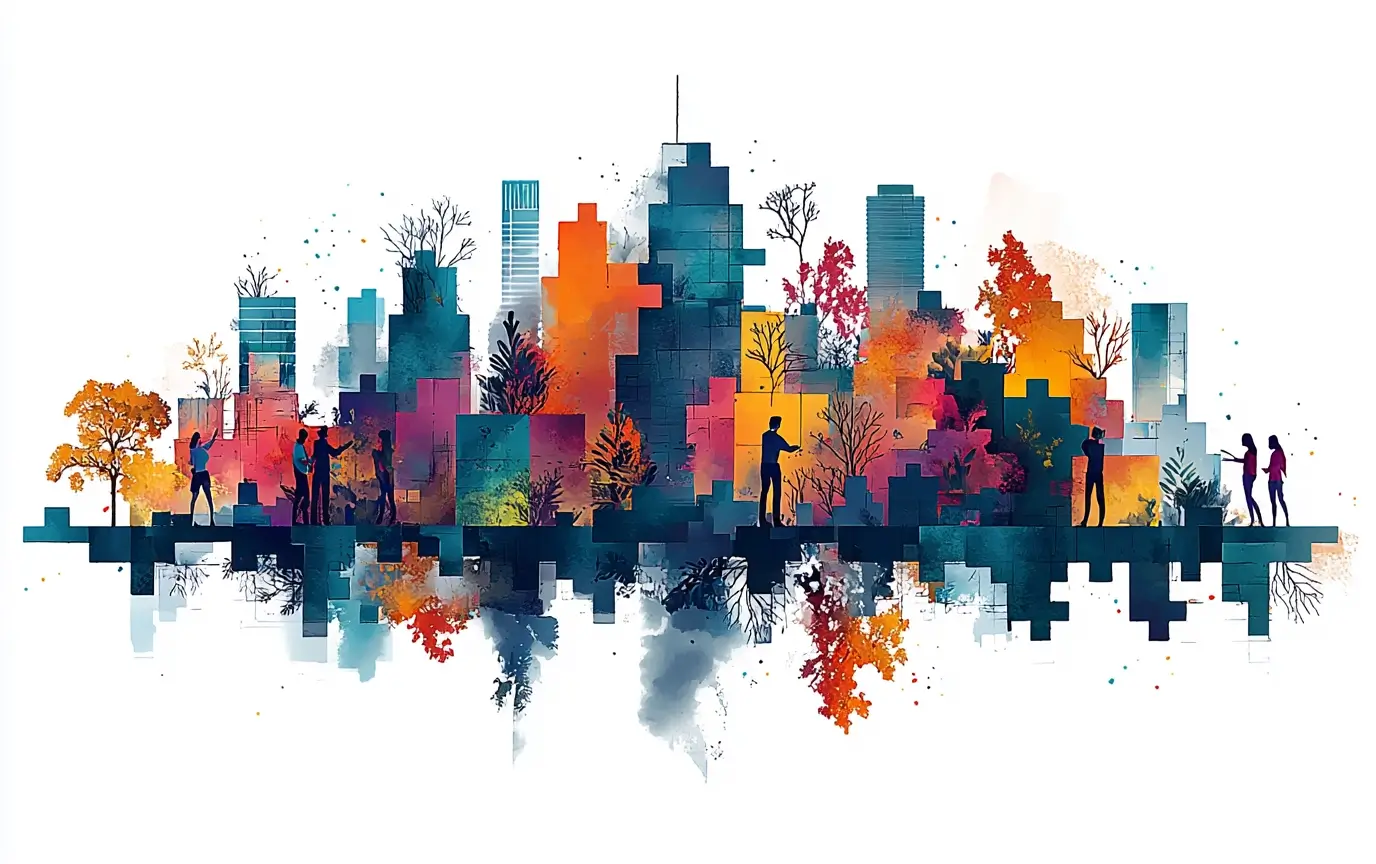
Why CX Starts With EX in 2026: Culture, Connection, Performance
%20Wheel.%20Mapping%20Outcomes.webp)
The Employee Experience (EX) Wheel: Mapping Outcomes

Behavioral Economics Can Best Be Described As "Psychology Meets Economics"

Behavioral Economics Is More Than Just Numbers
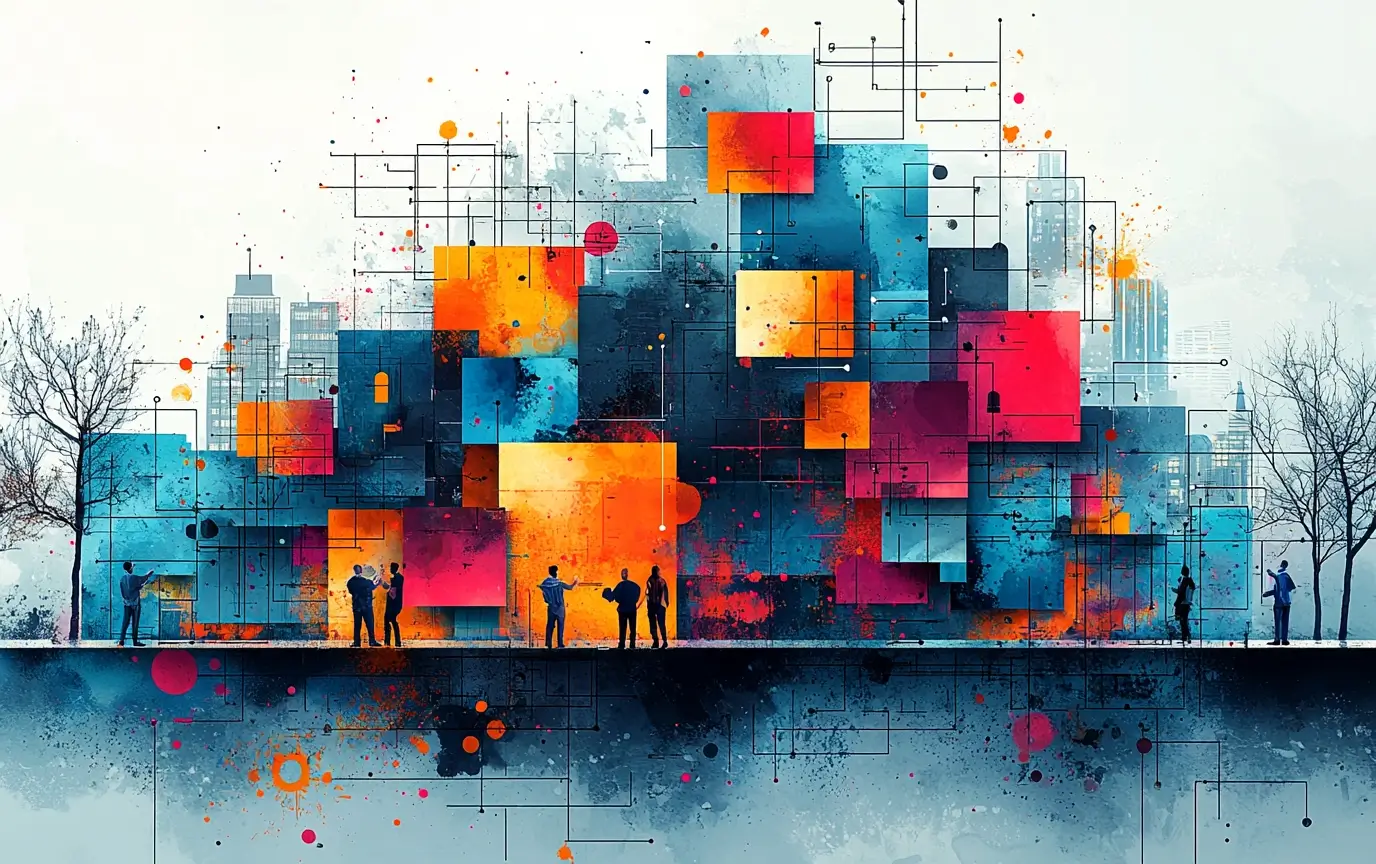
Behavioral Economics Explains Why People Are Irrational: And What to Do About It

Is Behavioral Economics Micro or Macro? Understanding Its Scope
%3F%20Strategies%20for%20Modern%20Organizations.webp)
How McKinsey Approaches Employee Experience (EX)? Strategies for Modern Organizations
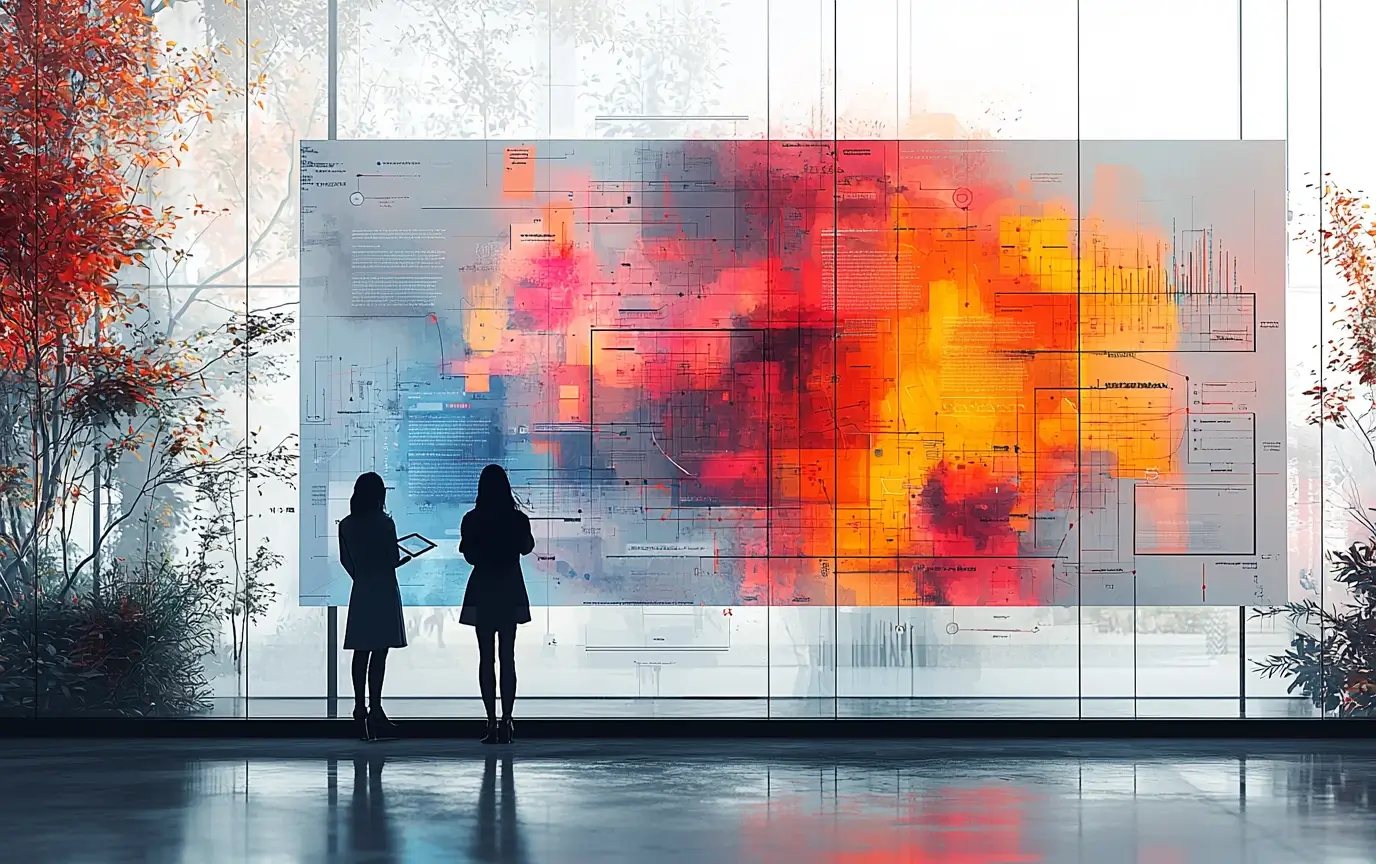
Behavioral Economics Is Dead: Debates on Its Future
%20Leader%20Do%3F.webp)
What Does an Employee Experience (EX) Leader Do?
%20Leader%20Do%3F.webp)
What Does an Employee Experience (EX) Leader Do?
%20Is%20Important%20in%202026.webp)