Customer Experience (CX) with AI: Enhancing the Customer Journey
%20with%20AI.%20Enhancing%20the%20Customer%20Journey.webp)
Artificial Intelligence (AI) is revolutionizing how businesses engage with customers, offering new ways to personalize interactions, streamline processes, and enhance the overall Customer Experience (CX). As AI technology continues to advance, its integration into CX strategies is becoming increasingly critical for companies looking to stay competitive. This article explores how AI is transforming CX, providing insights into key applications, case studies, and best practices for leveraging AI to enhance the customer journey.
1. Understanding the Role of AI in CX
AI plays a pivotal role in modernizing CX by automating processes, analyzing customer data, and delivering personalized experiences at scale. AI technologies such as machine learning, natural language processing, and predictive analytics enable businesses to understand and anticipate customer needs better than ever before.
Key Benefits:
- Personalization at Scale: AI allows businesses to deliver highly personalized experiences to millions of customers simultaneously, improving engagement and satisfaction.
- Efficiency and Automation: AI automates routine tasks, freeing up human agents to focus on more complex issues, which enhances the overall efficiency of customer service.
- Data-Driven Insights: AI-driven analytics provide deep insights into customer behavior, preferences, and pain points, enabling more informed decision-making.
Data Insight:
- CX Improvement: According to a study, companies that have integrated AI into their CX strategy have seen a 40% improvement in customer satisfaction and a 35% increase in operational efficiency.
Case Study:
- Netflix: Netflix uses AI to analyze viewer data and provide personalized content recommendations, resulting in higher viewer engagement and customer retention.
2. AI-Powered Personalization in CX
Personalization is a key driver of customer satisfaction, and AI enables businesses to take personalization to new heights. By analyzing vast amounts of data, AI can deliver tailored content, product recommendations, and communication at the individual level.
Applications of AI-Powered Personalization:
- Dynamic Content: AI algorithms can dynamically generate and display personalized content on websites, apps, and emails based on real-time customer data.
- Product Recommendations: AI analyzes past behavior and preferences to recommend products that are most likely to appeal to each customer, increasing conversion rates.
- Personalized Customer Journeys: AI helps create individualized customer journeys by predicting next best actions and offering personalized experiences at every touchpoint.
Data Insight:
- Conversion Boost: Personalization powered by AI can lead to a 20-30% increase in conversion rates and a 15-20% improvement in customer satisfaction.
Case Study:
- Amazon: Amazon’s recommendation engine, powered by AI, drives a significant portion of its sales by providing personalized product suggestions based on customer behavior and preferences.
3. Enhancing Customer Support with AI
AI is transforming customer support by enabling faster, more efficient, and more accurate responses to customer inquiries. AI-powered chatbots, virtual assistants, and automated response systems are now standard tools in the customer support arsenal.
Key AI Tools in Customer Support:
- Chatbots: AI-driven chatbots can handle a large volume of customer queries simultaneously, providing instant responses and resolving common issues without human intervention.
- Virtual Assistants: These AI tools can assist customers with complex inquiries by understanding natural language and providing relevant solutions or escalating the issue to a human agent.
- Automated Response Systems: AI can automate responses to routine customer queries, freeing up human agents to focus on more complex and high-value tasks.
Data Insight:
- Efficiency Gains: Implementing AI in customer support can lead to a 25% reduction in response times and a 30% increase in issue resolution rates.
Case Study:
- H&M: H&M uses AI-driven chatbots to handle customer inquiries, providing quick and accurate responses that improve the overall shopping experience.
4. AI-Driven Analytics and Predictive Insights in CX
AI-driven analytics provide businesses with the ability to predict customer behavior, identify trends, and uncover hidden insights from vast amounts of data. These predictive insights enable companies to proactively address customer needs and optimize the customer journey.
Key Applications:
- Customer Segmentation: AI can analyze customer data to create detailed segments based on behavior, preferences, and demographics, allowing for more targeted marketing and personalization.
- Churn Prediction: AI models can predict which customers are at risk of churning, enabling businesses to take proactive steps to retain them.
- Trend Analysis: AI-powered tools can identify emerging trends in customer behavior, allowing businesses to adapt their strategies in real-time.
Data Insight:
- Predictive Power: Companies using AI-driven analytics to predict customer behavior have seen a 30% increase in customer retention and a 25% improvement in marketing ROI.
Case Study:
- Spotify: Spotify leverages AI to analyze user behavior and preferences, providing personalized playlists and recommendations that keep users engaged and reduce churn.
5. AI and Customer Journey Mapping
Customer journey mapping is a critical component of CX strategy, and AI enhances this process by providing real-time insights and predictive analytics. AI-powered journey mapping tools help businesses visualize the customer journey, identify pain points, and optimize touchpoints for a smoother experience.
Enhancements through AI:
- Real-Time Mapping: AI enables real-time updates to customer journey maps, reflecting changes in customer behavior as they occur.
- Predictive Journey Paths: AI can predict potential customer paths based on historical data, allowing businesses to proactively address issues before they arise.
- Personalized Journey Optimization: AI can tailor journey maps for individual customers, ensuring that each interaction is optimized for their specific needs and preferences.
Data Insight:
- Journey Optimization: Businesses that use AI to enhance customer journey mapping see a 20% improvement in customer satisfaction and a 15% reduction in customer effort scores.
Case Study:
- Delta Airlines: Delta uses AI-powered journey mapping to optimize its customer experience, identifying pain points and streamlining the travel process for passengers.
6. The Role of AI in Enhancing Customer Loyalty Programs
AI is also transforming customer loyalty programs by providing personalized rewards, optimizing engagement strategies, and predicting future customer behavior. These advancements make loyalty programs more effective and engaging for customers.
AI Applications in Loyalty Programs:
- Personalized Rewards: AI analyzes customer data to offer personalized rewards that align with individual preferences, increasing engagement and satisfaction.
- Loyalty Program Optimization: AI can identify which aspects of a loyalty program are most effective, allowing businesses to refine their strategies for maximum impact.
- Predictive Engagement: AI can predict which customers are likely to disengage from a loyalty program and offer incentives to keep them active.
Data Insight:
- Increased Engagement: AI-driven loyalty programs see a 25% increase in customer engagement and a 20% improvement in customer retention.
Case Study:
- Sephora: Sephora uses AI to personalize its Beauty Insider loyalty program, offering tailored rewards and recommendations that have led to higher customer engagement and loyalty.
7. AI and Voice of Customer (VoC) Programs
Voice of Customer (VoC) programs are essential for gathering customer feedback and insights. AI enhances these programs by automating data collection, analyzing sentiment, and providing actionable insights.
AI Enhancements in VoC Programs:
- Automated Feedback Collection: AI tools can automatically collect and analyze customer feedback from multiple channels, including social media, surveys, and customer reviews.
- Sentiment Analysis: AI-driven sentiment analysis helps businesses understand the emotions behind customer feedback, providing deeper insights into customer satisfaction.
- Actionable Insights: AI can identify key themes and trends in VoC data, enabling businesses to make data-driven decisions to improve CX.
Data Insight:
- Feedback Utilization: Companies that integrate AI into their VoC programs see a 30% improvement in their ability to respond to customer feedback and a 25% increase in customer satisfaction.
Case Study:
- Coca-Cola: Coca-Cola uses AI-driven VoC programs to analyze customer feedback across various channels, helping the company quickly identify and address issues that impact the customer experience.
For more on leveraging AI in VoC programs, explore our Voice of Customer Services.
8. Ethical Considerations in AI-Driven CX
As AI becomes more prevalent in CX strategies, it’s important to consider the ethical implications of using AI to manage customer interactions. Businesses must ensure that their AI applications are transparent, fair, and respect customer privacy.
Key Ethical Considerations:
- Transparency: Customers should be aware when they are interacting with AI, and businesses should be transparent about how customer data is used.
- Bias and Fairness: AI models should be regularly audited to ensure they are not biased against any group of customers, and that they deliver fair outcomes for all.
- Privacy Protection: AI applications must comply with data privacy regulations, and businesses should prioritize customer consent and data security.
Data Insight:
- Customer Trust: Companies that prioritize ethical AI practices see a 20% increase in customer trust and a 15% improvement in customer satisfaction.
Case Study:
- Microsoft: Microsoft has implemented strict ethical guidelines for AI, ensuring transparency, fairness, and privacy in all AI-driven customer interactions.
9. Challenges in Implementing AI in CX
While AI offers significant benefits for CX, implementing AI solutions can be challenging. Businesses must overcome obstacles related to data quality, integration, and user adoption to fully realize the potential of AI.
Common Challenges:
- Data Quality: AI models rely on high-quality data to function effectively, and poor data quality can lead to inaccurate predictions and suboptimal CX outcomes.
- System Integration: Integrating AI with existing systems and processes can be complex, requiring significant time and resources.
- User Adoption: Employees may be resistant to adopting AI tools, particularly if they are perceived as replacing human jobs or adding complexity to workflows.
Strategies for Overcoming Challenges:
- Data Management: Invest in data management tools and processes to ensure that AI models are trained on accurate and relevant data.
- Phased Implementation: Introduce AI solutions gradually, starting with small-scale pilots and expanding as the technology proves its value.
- Employee Training: Provide training and support to help employees understand and embrace AI tools, emphasizing how AI can enhance rather than replace their roles.
Data Insight:
- Success Rates: Companies that take a strategic approach to AI implementation, addressing challenges upfront, see a 25% higher success rate in their AI-driven CX initiatives.
Case Study:
- Toyota: Toyota successfully implemented AI in its customer service operations by addressing data quality and integration challenges and providing comprehensive training for employees.
10. AI in CX for B2B vs. B2C Contexts
The application of AI in CX can differ significantly between B2B (business-to-business) and B2C (business-to-consumer) contexts. Understanding these differences is crucial for developing effective AI strategies tailored to each market.
B2B vs. B2C Differences:
- Customer Journeys: B2B customer journeys are often longer and more complex, requiring AI to manage multiple touchpoints and stakeholders. In contrast, B2C journeys are typically shorter and more transactional, allowing for more straightforward AI applications.
- Personalization Needs: B2B customers expect highly personalized interactions that address specific business needs, while B2C personalization focuses on individual preferences and convenience.
- Data Volume and Complexity: B2B companies often deal with smaller data sets but require deeper insights, while B2C companies manage large volumes of data that AI can analyze at scale.
Data Insight:
- AI Impact in B2B vs. B2C: B2B companies that use AI to manage complex customer journeys report a 20% increase in sales cycle efficiency, while B2C companies see a 30% improvement in customer engagement through AI-driven personalization.
Case Study:
- IBM (B2B): IBM uses AI to manage complex B2B customer journeys, providing personalized solutions that address the specific needs of its enterprise clients.
- Nike (B2C): Nike leverages AI to deliver personalized product recommendations and marketing campaigns, driving customer engagement and sales in the B2C market.
11. Measuring the Impact of AI on Customer Experience
To ensure that AI initiatives are delivering the desired outcomes, it’s essential to measure their impact on key CX metrics. This data provides insights into the effectiveness of AI applications and helps guide future investments.
Key Metrics:
- Customer Satisfaction (CSAT): Measure changes in CSAT scores to assess how AI-driven improvements are affecting overall customer satisfaction.
- Net Promoter Score (NPS): Track NPS to gauge how likely customers are to recommend your brand, a key indicator of loyalty and satisfaction.
- Customer Effort Score (CES): Evaluate how AI applications reduce customer effort in interactions, leading to smoother and more satisfying experiences.
- Operational Efficiency: Analyze metrics related to process efficiency, such as response times and issue resolution rates, to assess the impact of AI on operational performance.
Data Insight:
- Metric Correlation: High CSAT, NPS, and CES scores are strongly correlated with successful AI implementations in CX, leading to improved customer retention and revenue growth.
Case Study:
- Adobe: Adobe uses a combination of CSAT, NPS, and CES metrics to monitor and optimize the impact of AI on customer experience, resulting in higher customer satisfaction and loyalty.
12. The Future of AI in CX
As AI technology continues to evolve, its role in CX will expand, offering new opportunities for innovation and competitive advantage. Businesses that stay ahead of these trends will be better positioned to meet customer needs and maintain a competitive edge.
Future Trends:
- AI-Driven Personalization: AI will enable even more advanced levels of personalization, delivering highly customized experiences based on real-time data and predictive insights.
- AI and Augmented Reality (AR): The integration of AI with AR will create immersive, interactive experiences that enhance the customer journey, particularly in retail and entertainment.
- AI Ethics and Trust: As AI becomes more pervasive, businesses will need to prioritize ethical considerations and build customer trust by ensuring transparency, fairness, and privacy in AI applications.
Data Insight:
- Future Growth: Companies that invest in emerging AI technologies are expected to see a 35% increase in customer satisfaction and a 30% improvement in operational efficiency by 2025.
Case Study:
- Shopify: Shopify is leading the way in AI-driven CX innovation, using AI to power personalized shopping experiences, predictive analytics, and seamless customer support, resulting in significant improvements in customer engagement and sales.
13. Case Studies: Brands Leading in AI-Driven CX
Several brands stand out for their successful integration of AI into their CX strategies. These companies have leveraged AI to create seamless, personalized, and efficient customer experiences that drive loyalty and business growth.
Top Brands:
- Google: Google uses AI across its platforms to deliver personalized search results, targeted advertising, and responsive customer support, enhancing user satisfaction and engagement.
- Tesla: Tesla leverages AI in its customer service, using predictive analytics to anticipate customer needs and AI-powered tools to provide efficient support and personalized experiences.
- Starbucks: Starbucks uses AI to power its mobile app, offering personalized recommendations, rewards, and a seamless ordering experience that keeps customers coming back.
Data Insight:
- AI and CX Impact: Brands that excel in AI-driven CX achieve 2.5 times higher customer retention rates and 1.8 times higher revenue growth than their competitors.
Case Study:
- Nike: Nike’s AI-driven CX strategy, which includes personalized product recommendations, targeted marketing, and AI-powered customer support, has resulted in increased customer engagement and loyalty.
14. Final Thoughts: The Strategic Role of AI in Enhancing CX
AI is not just a tool for enhancing customer experience; it is a strategic imperative for businesses looking to stay competitive in the digital age. By leveraging AI to personalize interactions, streamline processes, and predict customer needs, companies can create exceptional experiences that drive satisfaction, loyalty, and long-term growth. As AI technology continues to advance, businesses that invest in AI-driven CX strategies will be well-positioned to lead in their industries, delivering value to both their customers and their bottom line.
Check Renascence's Signature Services
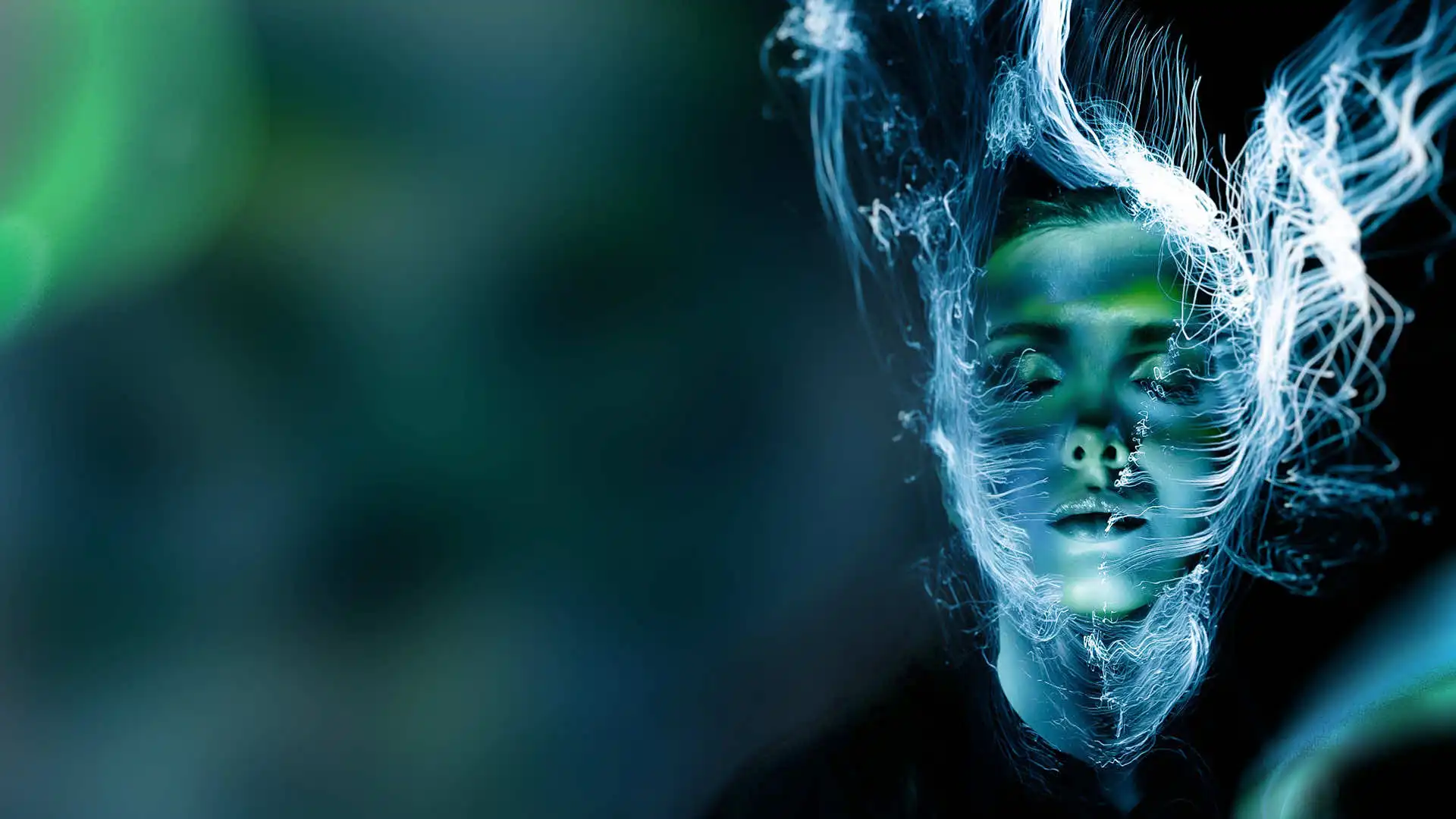
Behavioral Economics
Discover the power of Behavioral Economics in driving customer behavior.

Mystery Shopping
Uncover hidden insights with our mystery shopping & touchpoint audit services.
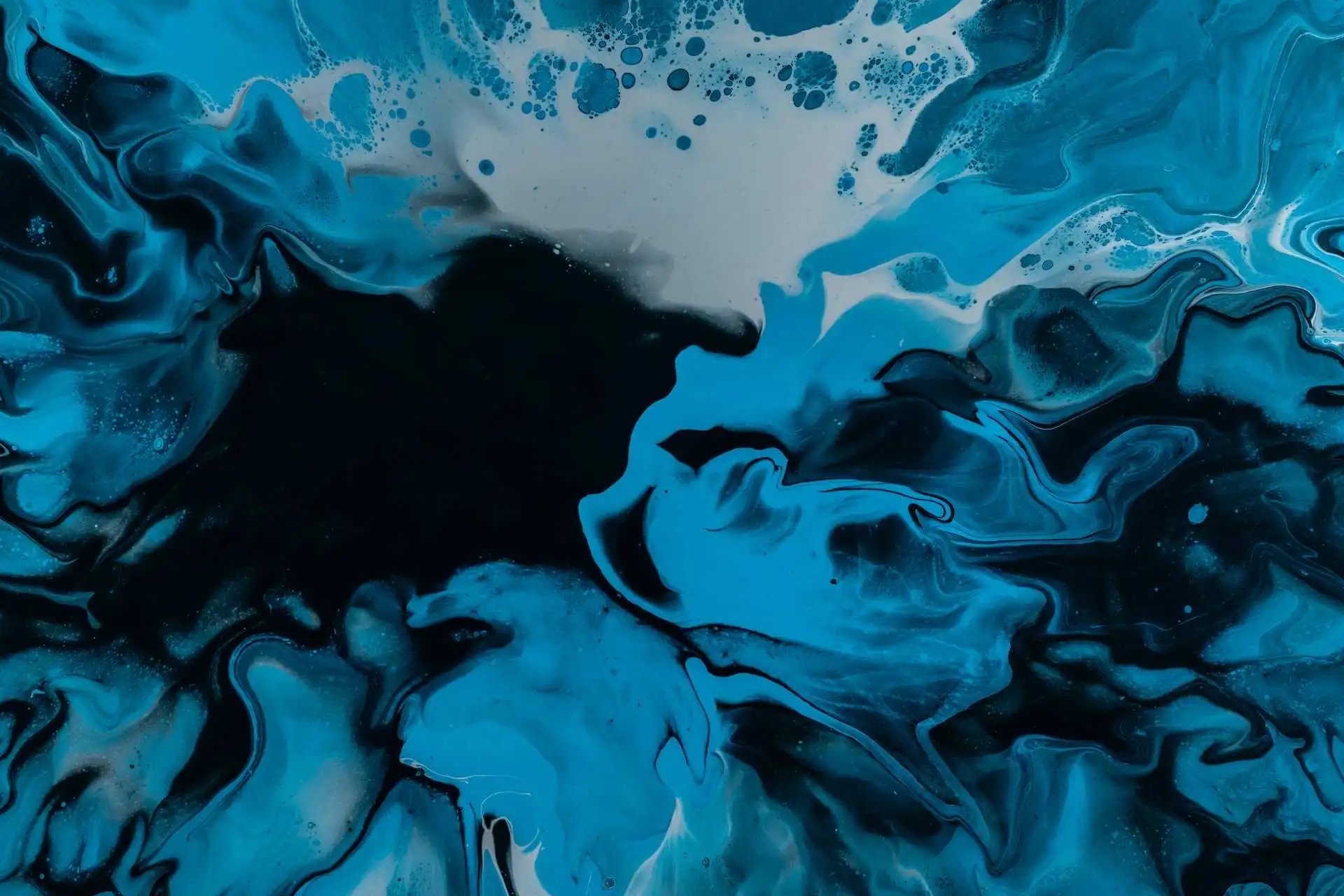
Experience Design
Crafting seamless journeys, blending creativity & practicality for exceptional experiences.
Experience Loom
Discover the latest insights from industry leaders in our management consulting and customer experience podcasts.
Aslan Patov x Gaia Living. Is Dubai's real estate market a bubble?
Experience Journal's Latest
Stay up to date with our informative blog posts.
%20Through%20Exceptional%20Dealership%20Services.webp)
How Bentley Elevates Customer Experience (CX) Through Bespoke Customization and Exceptional Dealership Services
%20with%20a%20Focus%20on%20Personalized%20Luxury.webp)
How Lexus Enhances Customer Experience (CX) with a Focus on Personalized Luxury and Technological Innovations
%20with%20Bespoke%20Customer%20Care.webp)
How Rolex Enhances Customer Experience (CX) with Timeless Craftsmanship and Bespoke Customer Care
%20with%20Exclusivity.webp)
How Hermès Delivers a Luxury Customer Experience (CX) with Exclusivity and Personalized Services
%20with%20Product%20Customization.webp)
How New Balance Enhances Customer Experience (CX) with Product Customization and Localized Marketing
%20with%20Personalized%20Shopping.webp)
How Adidas Innovates Customer Experience (CX) with Personalized Shopping and Sustainability Initiatives
%20with%20Digital%20Fitness%20Platforms.webp)
How Lululemon Elevates Customer Experience (CX) with Community Engagement and Digital Fitness Platforms
%20with%20Digital%20Insurance%20Solutions.webp)
How Allianz Improves Customer Experience (CX) with AI-Driven Claims and Digital Insurance Solutions
%20with%20Bespoke%20Luxury%20Services.webp)
How Rolls-Royce Delivers Exceptional Customer Experience (CX) with Bespoke Luxury Services
%20Through%20Community%20Engagement.webp)
How Harley-Davidson Builds Customer Experience (CX) Through Community Engagement and Brand Loyalty
%20with%20Immersive%20Dealership%20Technologies.webp)
How Audi Transforms Customer Experience (CX) with Immersive Dealership Technologies
%20with%20Personalized%20Dealership%20Services.webp)
How Mercedes-Benz Elevates Customer Experience (CX) with Luxury In-Car Technology and Personalized Dealership Services
%20with%20DT%20and%20Self-Service%20Solutions.webp)
How Beeline Improves Customer Experience (CX) with Digital Transformation and Self-Service Solutions
%20with%20AI%20and%20Personalized%20Telecom%20Services.webp)
How MTS Enhances Customer Experience (CX) with AI and Personalized Telecom Services
%20with%20Innovative%20Digital%20Services.webp)
How Three Mobile Enhances Customer Experience (CX) with Innovative Digital Services and Seamless Connectivity
%20with%20Smart%20Communities%20and%20Luxury%20Developments.webp)
How Emaar Elevates Customer Experience (CX) with Smart Communities and Luxury Developments
%20with%20Service%20Excellence%20%26%20Tech%20Innovation.webp)
How Qatar Airways Elevates Customer Experience (CX) with Luxury, Service Excellence, and Technological Innovation
%20with%20Luxurious%20In-Flight%20Services.webp)